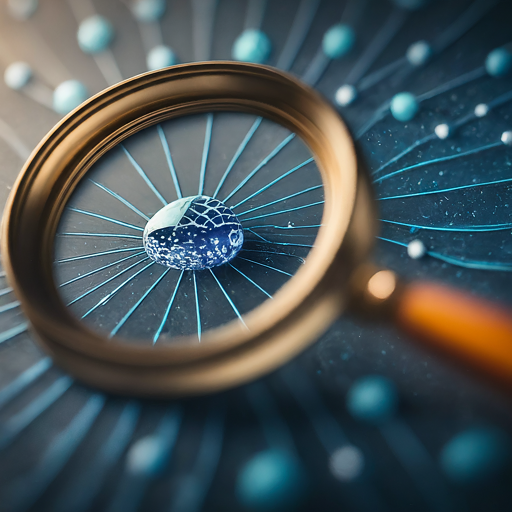
Navigating IoT Data Analytics: Overcoming Challenges for Enhanced Insights
In today’s digital age, the Internet of Things (IoT) has transformed the way we collect and analyze data. With billions of sensors and smart devices constantly generating information, organizations face formidable challenges in harnessing the power of this data deluge, particularly when it comes to data analytics within IoT systems.
Challenge 1: Data Volume and Variety
IoT devices generate massive amounts of data in different formats and from various sources, presenting challenges in storage and processing. To address this challenge, organizations need to invest in robust data preprocessing pipelines to clean, filter, and standardize IoT data. Additionally, scalable data storage solutions, such as cloud-based storage, can help accommodate the growing volume of IoT data. Leveraging advanced analytics techniques, like machine learning algorithms, can further extract meaningful insights from IoT data.
Challenge 2: Real-time Data Processing
Real-time data processing in IoT systems requires rapid analysis and action, which can be challenging due to high data velocities and latency requirements. To overcome this challenge, organizations can implement edge computing to process data locally and reduce latency. Stream processing frameworks, like Apache Kafka, can be utilized for real-time data ingestion and analytics. Additionally, deploying in-memory databases can help store frequently accessed data and reduce retrieval times.
Challenge 3: Interoperability of Data Flows
IoT ecosystems involve diverse devices and protocols, leading to interoperability challenges. To address this challenge, organizations should prioritize IoT devices that adhere to widely accepted open standards and communication protocols. Implementing middleware solutions to translate data formats and protocols can facilitate interoperability. Choosing IoT platforms that offer built-in support for diverse devices and protocols can further streamline data integration.
Challenge 4: Scalability of Data Infrastructure
As IoT ecosystems grow, traditional infrastructure may struggle to accommodate increasing data volumes and computational requirements. To ensure scalability, organizations can utilize distributed computing frameworks, like Apache Hadoop, to process large datasets across multiple nodes. Cloud-based IoT platforms and analytics services offer inherent scalability and flexibility. Adopting containerization technologies, such as Docker and Kubernetes, can enable modular and scalable architectures.
Challenge 5: Long-term Maintenance
IoT systems have extended lifecycles, requiring ongoing maintenance and updates. To tackle long-term maintenance issues, organizations should maintain comprehensive documentation of system architecture, hardware components, and software configurations. Implementing a robust update and patch management process for hardware and software components is essential. Designing IoT analytics systems with scalability and flexibility can accommodate future technological advancements.
By addressing these challenges with practical strategies, organizations can unlock the full potential of data analytics within their IoT ecosystems and drive innovation in various industries.